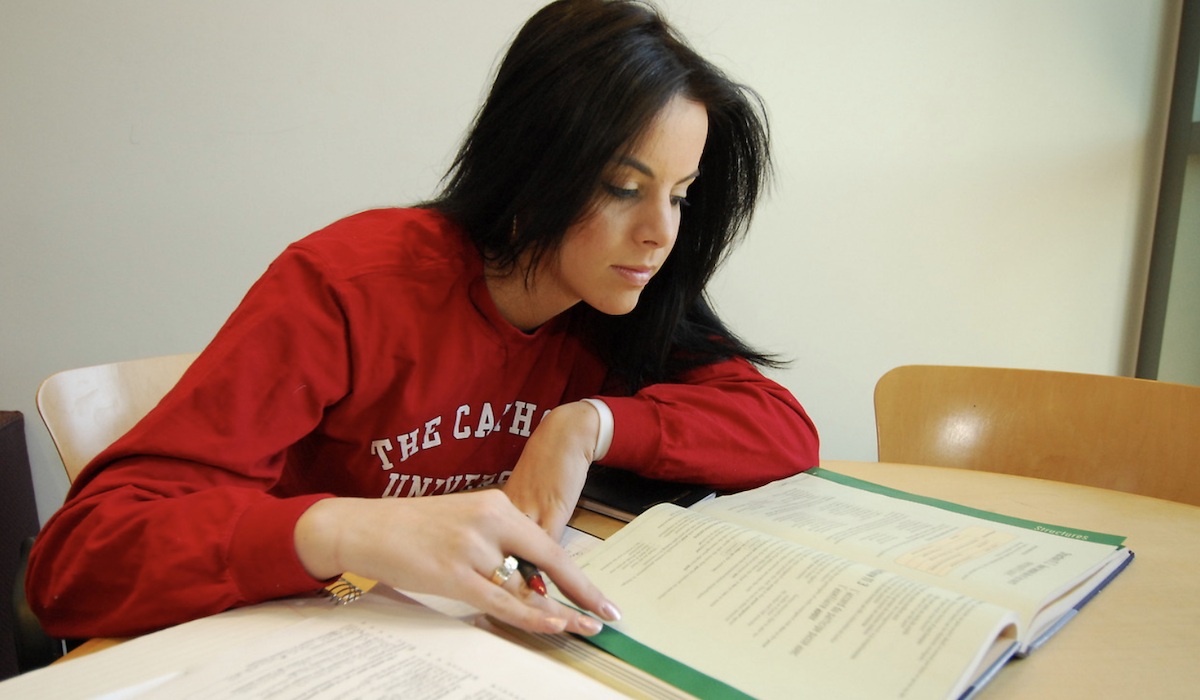
Assessment vs. Evaluation
In education, we often hear the terms assessment and evaluation used interchangeably. However, a deeper look reveals an important distinction, as highlighted by Banta & Palomba (2014), Huba & Freed (2000), and Maki (2010):
- Assessment is an ongoing process of gathering data through methods such as quizzes, assignments, and observations to provide feedback that improves teaching and learning. It is about monitoring student progress and adjusting teaching strategies to support learning outcomes better. For example, a professor might use weekly quizzes to gauge student understanding and adapt lesson plans accordingly.
- Evaluation is a systematic process of measuring the quality or value of student work, often resulting in a grade or certification. The goal is to judge overall performance, such as when a final exam or portfolio is used to determine whether students have mastered the course objectives.
Exploring the Role of AI
As AI technologies become more accessible, educators find themselves at a crossroads, wondering whether AI truly has a place in the classroom. While some embrace its potential to personalize feedback and assist with routine tasks like grading, others remain cautious, questioning how AI aligns with their pedagogical goals.
Does AI change what we want our students to learn? If AI can generate responses or help students analyze data, should we refocus our learning goals toward higher-order thinking skills, such as critical analysis, creativity, and ethical reasoning? And how does AI influence the way we approach assessment?
The challenge for educators is finding the right balance—using AI to complement, not replace, the meaningful interactions between instructors and students. AI can offer assistance, but the educator’s role is to guide and assess deeper learning.
Guaranteeing the Authenticity of Student Work
With the increasing use of AI tools, ensuring the authenticity of student work has become a growing concern. Educators must design assessments that encourage original thought and minimize the risk of AI misuse. Clear communication about AI’s role in the classroom, reinforced through syllabus policies, can help guide students in understanding when and how AI tools are permitted. As outlined by The Busch School of Business in the Initial Recommendations on Generative Artificial Intelligence, these policies can include unrestricted, guided, or restricted use of AI, depending on the course’s objectives and the instructor's preferences (Mohanan, Pak, Pakaluk, Torres, Wall, & Yoest, 2024).
Additionally, educators can design AI-proof assessments by incorporating components that are difficult for AI to replicate, such as oral assessments, real-world applications, or projects that require personalized responses. These assessments challenge students to demonstrate their understanding and apply their knowledge in unique contexts, ensuring that their work reflects authentic learning and not simply AI-generated content. Testing knowledge orally or requiring students to provide explanations for their answers can help safeguard against over-reliance on AI tools.
The Unified Whole of Teaching
In their book Learning Assessment Techniques: A Handbook for College Faculty, Barkley and Major (2016) present a framework for effective teaching centered around three interconnected elements: Learning Goals, Learning Activities, and Outcomes Assessment. These elements work together, forming a unified whole in which adjustments to one inevitably affect the others.
This interconnectedness becomes even more important in the age of AI. As we adapt our learning goals to reflect the skills our students need in a rapidly changing world, our assessment methods must evolve accordingly.
Learning Assessment Techniques (LATs)
One structured approach that helps educators navigate these evolving demands is the Learning Assessment Techniques (LATs) cycle. Introduced by Barkley and Major (2016), LATs offer a flexible yet comprehensive method for assessing student learning across various contexts. A key component of the LAT framework is its grounding in Fink’s Taxonomy of Significant Learning, which emphasizes six interconnected dimensions beyond simply recalling facts or skills. These dimensions include Foundational Knowledge, Application, Integration, Human Dimension, Caring, and Learning How to Learn (Fink, 2013).
Fink’s taxonomy encourages educators to think more holistically about learning by incorporating leadership, ethics, interpersonal skills, and character development into their teaching. By using LATs, educators can design assessments that foster meaningful learning. For example, while AI might help assess factual recall (Foundational Knowledge), human judgment is still essential for evaluating reflective activities that challenge students to apply learning to real-world contexts (Application) or consider the ethical implications of a decision (Human Dimension).
The six steps in the LAT cycle align well with Fink’s holistic approach to learning:
- Clarify what you want students to learn: Identify the specific knowledge, skills, or attitudes students should acquire, aligning with Fink's domains. Professors can use the Learning Goals Inventory from Barkley and Major’s handbook to pinpoint relevant learning goals and the appropriate domain.
- Determine why you are assessing their learning: Decide if the goal is formative (to provide feedback and guide student learning) or summative (to evaluate and assign a grade).
- Select a LAT: Choose one of the 50 LATs discussed by Barkley and Major (2016) that aligns with your learning goals. For example, Guided Reading Notes help assess foundational knowledge, while the What? So What? Now What? Journal encourages students to reflect on their learning process.
- Implement the LAT in your course: Introduce the LAT, explaining its purpose and the expected outcomes to ensure students are fully engaged.
- Analyze and report the results: Use rubrics or other tools to evaluate student work, providing clear feedback on areas of strength and opportunities for improvement.
- Evaluate and make changes: Reflect on the results and consider how to refine the LAT or adjust your learning goals for future iterations.
By following the LAT cycle, educators can tailor their assessment methods to diverse needs while retaining the flexibility to integrate AI tools where appropriate. Fink’s taxonomy provides a useful framework for understanding significant learning, helping students not just memorize facts but develop a deeper understanding that they can apply, connect to their lives, and use to continue learning.
Resources for Faculty
For faculty interested in learning more about Learning Assessment Techniques or thoughtfully integrating AI into their assessments, we encourage you to access materials from our recent presentations (CUA sign-in required):
- Approaches to Assessment and Evaluation in the Age of Artificial Intelligence: Session Recording + Slideshow
- Learning Assessment Techniques: Session Recording + Slideshow + Summary of the 50 Techniques
Additionally, copies of Learning Assessment Techniques by Barkley and Major (2016) are available through the CTE Library and the Catholic University Libraries.
As AI continues to influence education, how we approach assessment and evaluation will play a critical role in shaping student learning. By leveraging frameworks like LATs and exploring AI's potential mindfully and critically, we can ensure that our assessments remain aligned with our learning goals and foster meaningful, long-term student growth.
References
Banta, T. W., & Palomba, C. A. (2014). Assessment essentials: Planning, implementing, and improving assessment in higher education. John Wiley & Sons. (CUA Library Permalink)
Barkley, E. F., & Major, C. H. (2016). Learning assessment techniques: A handbook for college faculty. John Wiley & Sons. (CUA Library Permalink)
Fink, L. D. (2013). Creating significant learning experiences: An integrated approach to designing college courses. San Francisco: Jossey-Bass. (CUA Library Permalink)
Huba, M. E., & Freed, J. E. (2000). Learner-centered assessment on college campuses: Shifting the focus from teaching to learning. Allyn & Bacon. (CUA Library Permalink)
Maki, P. L. (2010). Assessing for learning: Building a sustainable commitment across the institution. (2nd ed.). Sterling, VA: Stylus Publishing, LLC. (CUA Library Permalink)
Mohanan, G., Pak, C., Pakaluk, M., Torres, M., Wall, L., & Yoest, J. (2024, February). Initial recommendations on generative artificial intelligence. Ad-Hoc A.I. Committee. The Busch School of Business. The Catholic University of America. (Available to CUA users via Google Drive.)
__________
Sarah Radice, M.S., M.A., is an instructional designer at CTE. As a versatile learning and development specialist with a proven track record in instructional design and teaching, she offers 15+ years of experience in developing effective learning solutions.
Diego A. Boada, Ph.D., EMBA, is an instructional designer at CTE. He specializes in finding scalable solutions for learning and performance problems in multicultural environments. Dr. Boada has designed online learning experiences for universities, startups, and organizations globally; consulted for the Inter-American Development Bank; and collaborated with Silicon Valley leaders.